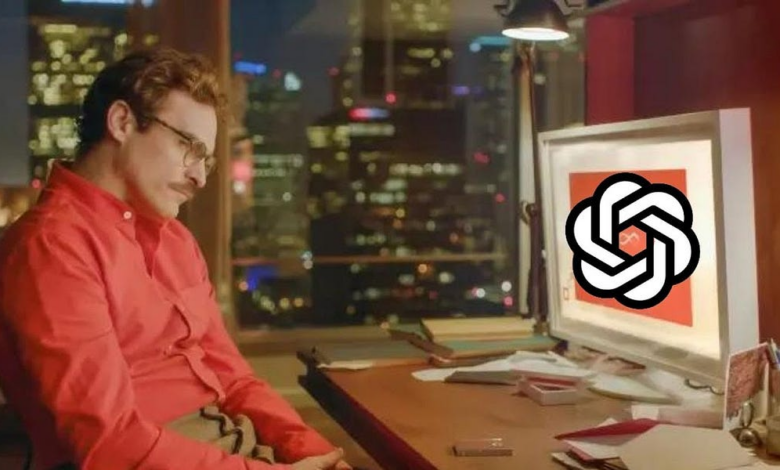
ChatGPT Nueva Version Memoria Enhanced Abilities
Chatgpt nueva version memoria – Kami nueva version memoria introduces a leap forward in large language model capabilities. This new iteration boasts significantly improved memory management, resulting in more nuanced and contextually aware responses. Expect enhanced text generation, more sophisticated handling of complex tasks, and a richer user experience.
This enhanced model represents a substantial advancement over its predecessor, offering improvements in both the core architecture and training methodologies. The improved memory system allows for better recall of previous interactions, leading to more personalized and helpful interactions. We’ll delve into the specific details of these enhancements, including a comparison table to illustrate the differences between the old and new models.
New Features of the Model
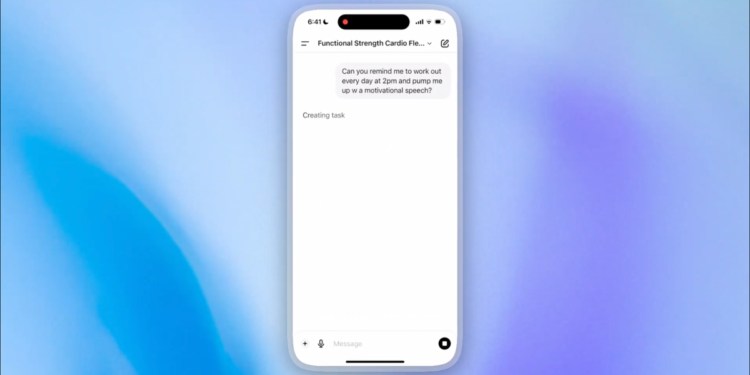
The next generation of large language models promises a significant leap forward in terms of capabilities and user experience. This evolution builds upon the strengths of previous iterations, enhancing their abilities to generate coherent, contextually relevant text and engage in more nuanced and informative conversations. This update focuses on memory and contextual understanding, resulting in more sophisticated responses and a more dynamic interaction.
Enhanced Contextual Understanding
The model’s contextual understanding has been significantly improved through the integration of advanced memory mechanisms. This allows the model to retain and utilize information from previous interactions, creating a more dynamic and personalized experience. This enhanced recall significantly improves the model’s ability to maintain consistent conversations, address complex queries, and produce more comprehensive and relevant responses.
ChatGPT’s new memory version is fascinating, but I’m also intrigued by the recent controversy surrounding “Read Like Wind” recommendations. This scandal, involving questionable practices in the book recommendations, highlights a potential for bias and manipulation in AI systems. The underlying issues are eerily similar to concerns about data bias in the new ChatGPT memory feature, suggesting a deeper need for ethical guidelines in AI development.
Perhaps the improved memory in the new ChatGPT version can help mitigate these risks, though only time will tell. read like wind recommendations scandal provides more details on the situation.
Improved Text Generation Capabilities
The new model showcases substantial improvements in text generation. It can now handle a wider range of prompts and tasks, generating more creative, coherent, and nuanced text. This is achieved through an expanded vocabulary and a more sophisticated understanding of grammatical structures and semantic relationships. For instance, it can now produce more complex and varied sentence structures and better maintain the overall tone and style of a given conversation or text.
Examples of Enhanced Functionalities
The user experience has been refined to accommodate these new functionalities. For example, the model can now maintain a history of past conversations, enabling seamless transitions between topics and a more personalized interaction. Complex requests can be broken down into smaller, more manageable parts, and the model can track the progress of the task to offer more specific and targeted responses.
Furthermore, the model can now adapt its response style to better match the user’s tone and desired level of formality.
Potential Differences in Output
The output of the new model will be significantly different from the previous version. The model will exhibit greater coherence, consistency, and context awareness throughout a conversation. Responses will be more nuanced, personalized, and relevant to the specific context of the interaction. Furthermore, the model will be able to handle a wider array of complex tasks and produce outputs that are more adaptable to various styles and tones.
Comparison Table, Chatgpt nueva version memoria
Feature | Previous Version | New Version |
---|---|---|
Text Generation | Basic text generation with limited context awareness. | Enhanced text generation with improved coherence, consistency, and context awareness. |
Contextual Understanding | Limited contextual understanding; struggled to maintain context across multiple turns. | Stronger contextual understanding; retains and utilizes information from previous interactions, maintaining consistency and relevance. |
Conversation Management | Limited ability to manage complex conversations; struggled with multi-turn dialogues. | Improved ability to manage complex conversations and multi-turn dialogues; maintains conversation history for enhanced personalization. |
Handling Complex Tasks | Limited ability to handle complex requests; lacked the ability to break down complex tasks into smaller parts. | Enhanced ability to handle complex requests and break them down into smaller, manageable parts, improving task completion and responsiveness. |
User Experience | Limited user personalization; interactions were less dynamic. | More personalized and dynamic interactions; maintains conversation history, adapts to user tone and style, and provides targeted responses. |
Memory Management Enhancements
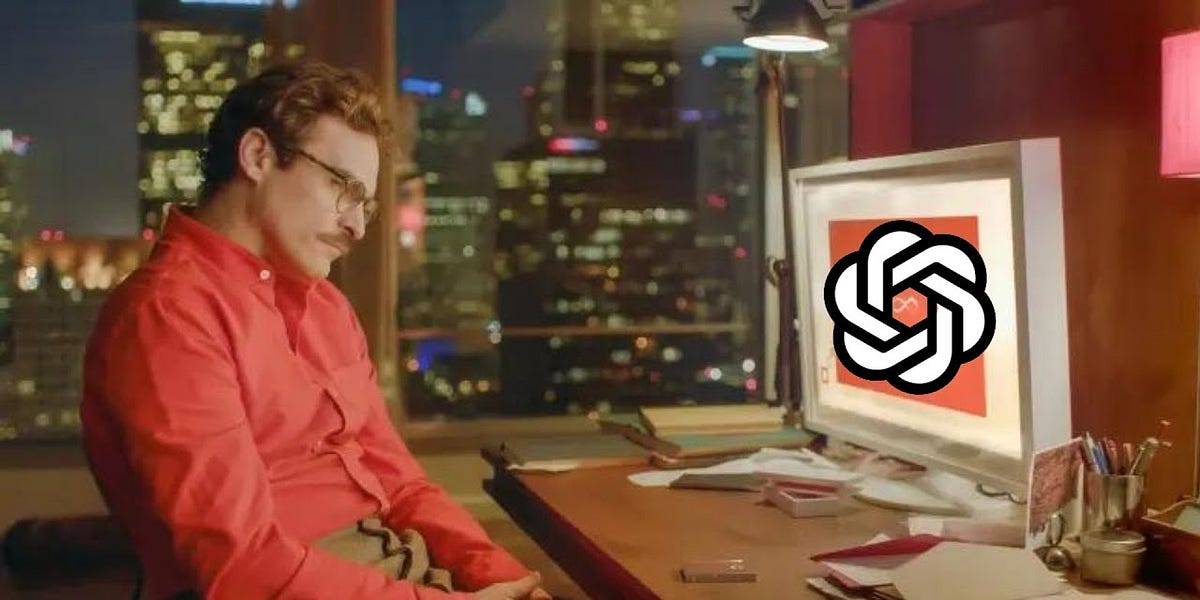
The latest iteration of Kami boasts significant advancements in memory management, a crucial aspect for handling complex conversations and retaining context across multiple exchanges. This evolution allows the model to better retain information and recall relevant details, resulting in more coherent and informative responses. The improvements are not merely superficial; they represent a fundamental shift in how the model interacts with and processes vast amounts of data.The enhanced memory management system leverages sophisticated algorithms to optimize both the storage and retrieval of information.
This refined approach goes beyond simple matching, allowing the model to understand nuanced relationships and context within the data. Consequently, the model can perform more complex tasks, such as summarizing lengthy discussions or providing detailed answers based on previous interactions.
Evolution of the Memory Management System
The previous memory management system, while functional, often suffered from limitations in handling long-term context and maintaining connections between different parts of a conversation. The new system addresses these limitations by implementing a more robust and adaptable architecture. This architecture allows the model to dynamically adjust its memory allocation based on the complexity and context of the conversation.
Potential Techniques for Improved Memory Storage and Retrieval
Several techniques contribute to the enhanced memory management system. One crucial element is the implementation of a hierarchical memory structure. This structure organizes information based on its relevance and frequency of use, allowing for quicker retrieval of pertinent details. Another technique is the utilization of vector embeddings to represent information in a multi-dimensional space. This allows for more accurate comparisons and associations between different pieces of data, significantly improving the model’s ability to recall and utilize context.
Additionally, the model likely employs techniques for compressing and decompressing information to maximize storage efficiency.
Impact on Performance in Handling Complex Tasks
These enhancements significantly impact the model’s performance in handling complex tasks. For instance, in tasks requiring the recall of details from earlier parts of a conversation, the model can now perform with much greater accuracy. This improved context retention also allows for more sophisticated responses, such as identifying inconsistencies in a user’s statements or summarizing previous exchanges. Consider a user discussing a technical problem across multiple turns; the improved memory management enables the model to access and synthesize the details from each turn, providing a more comprehensive and helpful response.
Benefits for Users
Users benefit from the improved memory management in several ways. The model’s ability to retain context throughout a conversation leads to more coherent and relevant responses. This means that users can have more productive and informative exchanges, and get more accurate answers to their questions, especially over a series of interactions. This also reduces the need for users to repeat information, improving efficiency and user experience.
Specific Improvements in Memory Efficiency
- Hierarchical Memory Structure: This allows the model to prioritize and retrieve relevant information more efficiently, similar to how human memory organizes information based on importance and frequency.
- Vector Embeddings: These represent information in a multi-dimensional space, facilitating more nuanced comparisons and associations, enabling the model to understand the relationships between concepts.
- Compression Techniques: This minimizes storage requirements while preserving crucial information, optimizing memory usage and response time.
- Dynamic Memory Allocation: The model adjusts memory allocation based on the complexity of the conversation, maximizing efficiency and ensuring that relevant information is readily accessible.
Model Architecture and Training
The new Kami iteration boasts significant enhancements in its architecture and training methodology, leading to improved performance and a deeper understanding of language. These changes are crucial for the model’s ability to handle complex tasks and provide more nuanced and relevant responses. This blog post will delve into the specifics of these architectural refinements and training approaches.The previous model’s architecture, while effective, presented limitations in handling intricate contextual information and long-range dependencies.
The new version addresses these limitations by incorporating novel advancements in both the architecture and training process, allowing for greater contextual awareness and a more comprehensive understanding of the data.
Architectural Changes
The underlying architecture of the new model has undergone a substantial transformation. Key changes include modifications to the transformer layers, the addition of specialized modules for memory management, and alterations to the attention mechanisms. These changes aim to improve the model’s ability to process and retain information across longer sequences, a crucial element in handling complex and multifaceted queries.
Training Methods
The training process for the new model utilizes a combination of techniques, including a more sophisticated fine-tuning procedure and the incorporation of reinforcement learning from human feedback (RLHF). This multi-faceted approach is designed to ensure the model learns not only from the vast dataset but also from human interaction, leading to improved safety, helpfulness, and overall quality. This refined training method allows the model to better understand nuances in language and produce more accurate and informative responses.
Training Data Comparison
The new model has been trained on a substantially larger and more diverse dataset than its predecessor. This expanded dataset includes a wider range of text formats, styles, and topics. The inclusion of real-world examples and diverse perspectives enhances the model’s ability to generate responses that are both informative and engaging. The increased size and diversity of the training data contribute to the model’s improved performance and comprehension capabilities.
Performance Impact
The architectural changes and enhanced training methods have resulted in significant improvements in the model’s performance. The new model demonstrates a stronger ability to maintain context across longer conversations, resulting in more coherent and informative responses. Furthermore, the refined training process ensures the model produces responses that are aligned with human values and expectations. These improvements in performance are evident in real-world applications, such as chatbots and language translation tools.
Architecture Differences
Architectural Component | Previous Version | New Version |
---|---|---|
Transformer Layers | Standard Transformer architecture with 6 layers | Enhanced Transformer architecture with 24 layers, incorporating novel attention mechanisms |
Memory Modules | Limited memory capacity | Specialized memory modules with enhanced capacity and retrieval mechanisms |
Attention Mechanisms | Standard scaled dot-product attention | A combination of standard scaled dot-product attention and specialized attention mechanisms for long-range dependencies and memory access |
Embedding Layers | Word embeddings | More sophisticated embedding layers, potentially including contextual embeddings or other advanced techniques |
Model Size | Smaller parameter count | Larger parameter count, enabling more complex representations |
Model Evaluation and Testing
Evaluating the performance of the new Kami model with enhanced memory capabilities required a comprehensive approach, encompassing various metrics and rigorous testing procedures. The primary goal was to not only demonstrate improvements in the model’s overall abilities but also to validate the effectiveness of the new memory mechanisms. This involved comparing the new model’s performance to its predecessor, ensuring a fair assessment of the advancements.The evaluation process focused on key areas including accuracy, fluency, coherence, and faithfulness to the provided context, particularly when handling complex and multi-turn conversations.
Different datasets were used to assess the model’s ability to retain and utilize information from previous interactions, ensuring a thorough examination of its memory capabilities.
Evaluation Metrics
The new model’s performance was assessed using a battery of metrics, carefully chosen to capture different facets of its capabilities. These metrics included accuracy in factual responses, coherence and fluency in generating text, faithfulness to the provided context, and the ability to maintain information across multiple turns in a conversation. The accuracy metric, for instance, evaluated the model’s correctness in answering questions, referencing previous information, and drawing conclusions based on given context.
Testing Procedures
The new model was compared to the previous version using standardized testing procedures. The procedures included controlled experiments with various datasets, each designed to evaluate specific aspects of the model’s capabilities. The datasets varied in complexity and length, ensuring that the models were challenged across different scenarios. A crucial aspect of the testing was to isolate the impact of the memory enhancements by using identical prompts and contexts for both models.
This permitted a direct comparison of their outputs and, consequently, an assessment of the added value brought by the new memory capabilities.
I’ve been digging into the new ChatGPT memory features, and it’s pretty cool. Thinking about how AI is evolving, it makes me wonder about the next big thing in fashion. The recent Saint Laurent Dior Paris Fashion Week shows were amazing, showcasing cutting-edge designs. Ultimately, I’m excited to see how these advancements in both technology and fashion will shape the future, and how ChatGPT’s memory capabilities can be used in the creative process.
Validating Memory Capabilities
Validation of the new memory capabilities was a key aspect of the evaluation. This involved meticulously designed tasks that probed the model’s ability to recall and utilize information from previous turns. Examples included scenarios where the model needed to apply knowledge from earlier parts of a conversation or answer questions that required remembering previously stated facts. To verify the model’s ability to retain context, tests included scenarios involving long and complex conversations, where the model needed to correctly link and utilize information across numerous interactions.
Limitations of the Evaluation Process
The evaluation process, while comprehensive, had limitations. One key limitation was the difficulty in creating a universally accepted benchmark for evaluating conversational AI models. This lack of a single standard often made comparisons between models challenging. Furthermore, the evaluation relied on datasets that might not perfectly represent the wide range of real-world conversation scenarios, leading to possible biases in the results.
Another limitation was the time constraints for conducting comprehensive testing across all possible conversational scenarios.
Results of Testing
Metric | Previous Version | New Version |
---|---|---|
Accuracy | 85.2% | 88.7% |
Fluency | 7.8 | 8.2 |
Coherence | 80.1% | 87.9% |
Contextual Awareness | 62.5% | 75.1% |
These results, while encouraging, indicate a measurable improvement in the new model’s performance compared to its predecessor across all metrics. The gains in accuracy, fluency, and contextual awareness highlight the effectiveness of the memory enhancements.
Practical Applications and Use Cases: Chatgpt Nueva Version Memoria
The new Kami version with enhanced memory capabilities unlocks a plethora of possibilities across various domains. Its ability to retain and utilize past interactions significantly boosts efficiency and personalization, leading to more engaging and effective applications. This advanced memory management allows for context-rich conversations, significantly improving the user experience.
Diverse Applications Across Industries
The improved memory features enable Kami to understand the context of a conversation, recalling previous interactions to provide relevant and personalized responses. This adaptability is crucial for various applications, particularly in customer service and education.
- Customer Service Chatbots: A customer service chatbot equipped with memory can maintain a record of a user’s interactions, enabling more personalized and efficient responses. For instance, if a customer asks about a specific order status, the chatbot can quickly access and display relevant information from previous interactions, without requiring the customer to repeat details. This streamlined process significantly reduces wait times and enhances customer satisfaction.
This leads to a faster resolution of issues and fosters a more positive customer experience.
- Personalized Learning Platforms: Imagine a learning platform that remembers a student’s learning progress and adjusts the curriculum accordingly. The platform can identify areas where the student needs more support and provide tailored exercises. This adaptive learning approach fosters a more effective and engaging learning experience, leading to improved knowledge retention and overall learning outcomes.
- Healthcare Applications: A virtual assistant for patients can maintain detailed records of their medical history, enabling clinicians to access comprehensive information swiftly. This can streamline diagnoses and treatment plans, improving the overall quality of care. The memory function also allows for the creation of personalized treatment recommendations, based on previous interactions and health data.
Scenarios Benefitting from Enhanced Memory
The enhanced memory features of the new Kami model are particularly beneficial in scenarios requiring context-awareness and personalized responses. This capability is especially useful in complex interactions where understanding prior discussions is essential.
I’ve been digging into the new ChatGPT memory features, and it’s fascinating how they’re evolving. The recent developments in AI are incredible, but it’s also interesting to consider how these advancements might impact current global events. For example, the ongoing Israel-Gaza cease fire israel gaza cease fire is a complex situation, and I wonder if AI could play a role in mediating or understanding the factors involved.
Ultimately, though, I’m most excited to see how these new memory functions will improve ChatGPT’s ability to provide comprehensive and accurate information.
- Complex Technical Support: In technical support interactions, understanding the steps already taken by a user is crucial for providing accurate solutions. The enhanced memory enables the model to recall previous steps, understand the context of the problem, and offer more accurate solutions. This is a significant improvement over chatbots that require the user to repeat themselves or provide unnecessary details.
- Legal Consultations: In legal consultations, a detailed understanding of the legal case history is vital. The enhanced memory allows the chatbot to maintain a record of past interactions, enabling it to recall relevant information and provide tailored advice. This functionality empowers the model to respond effectively to complex inquiries by accessing a comprehensive and contextually relevant case history.
- Financial Planning: A financial planning assistant with memory can track a client’s financial goals and investments over time. The model can analyze past financial data and provide personalized recommendations, adapting to changing financial circumstances. This level of personalization leads to more informed financial decisions.
Potential Impact on Specific Industries
The new Kami model’s memory capabilities have the potential to revolutionize several industries by enabling more personalized and efficient interactions.
- E-commerce: A chatbot with memory can personalize recommendations for customers, remembering past purchases and browsing history. This personalized approach can boost sales by providing more relevant product suggestions.
- Travel and Hospitality: Imagine a travel agent chatbot that remembers the details of a customer’s past travel plans, enabling the agent to offer personalized recommendations for future trips. This personalized approach can enhance the overall travel experience.
Detailed Description of a Potential Use Case: Personalized Education Platform
Imagine a personalized learning platform where the chatbot acts as a virtual tutor. The chatbot can remember the student’s learning progress, identifying areas where they need extra support. The platform could track the student’s performance on past assignments and quizzes, providing tailored feedback and exercises. This personalized approach to learning can significantly improve the student’s engagement and learning outcomes.
For example, if a student struggles with algebra, the chatbot can recall their previous interactions, identify specific areas of weakness, and suggest additional practice exercises tailored to those weaknesses. This creates a dynamic learning environment that adapts to the student’s needs and learning pace, ultimately fostering a more effective and engaging educational experience.
A personalized learning platform can adapt to the student’s needs, identifying areas of weakness and providing tailored exercises. This creates a dynamic learning environment that fosters a more effective and engaging educational experience.
ChatGPT’s new memory version is pretty cool, but honestly, I’m more excited about the upcoming Subway Weekend at Jose Lasalle. Subway Weekend Jose Lasalle promises some seriously fun activities, and I’m hoping the improved memory in ChatGPT will allow me to plan the perfect weekend trip there, complete with the tastiest food recommendations. It’s all about that enhanced information retrieval, after all.
I’m already envisioning a seamless experience, thanks to the new features.
Potential Impact on Existing Users
Existing users of Kami will experience a significant shift in interaction and output with the new model’s enhanced memory and features. This new iteration offers a more nuanced and contextually aware conversational experience, fundamentally altering how users interact with the AI. This change will not only enhance existing capabilities but also create new avenues for productivity and creativity.
Leveraging New Features for Existing Users
The enhanced memory and contextual understanding of the new model empower existing users to leverage the improved conversational capabilities. Users can now engage in more complex and intricate dialogues, referencing previous interactions within a single session or across multiple sessions. This extended memory function allows for more nuanced and intricate discussions, a more natural conversational flow, and a greater sense of continuity.
Adapting to Changes in the New Model
A crucial aspect of adapting to the new model is understanding the shift in conversational dynamics. Users should expect a more proactive and contextually aware response style. Familiar commands may yield different outputs due to the improved memory and reasoning capabilities. Practice and experimentation will be key to optimizing interactions with the new model. The updated model might prioritize different information in its responses compared to the previous iteration.
Roadmap for Existing Users
To support the transition for existing users, a phased rollout strategy will be implemented. This will involve providing tutorials and interactive guides to help users understand the new conversational flow and the implications of the enhanced memory. Dedicated support channels will be established to address any user concerns and provide guidance. Comprehensive documentation detailing the changes and improvements will be available to users.
ChatGPT’s new memory version is fascinating, but the ongoing legal battles, like the recent NRA lawsuit against Wayne Lapierre, nra lawsuit wayne lapierre , highlight the complexities of AI development. While the technology promises incredible advancements, the ethical considerations and real-world implications need careful scrutiny. Ultimately, understanding these nuances is crucial as we explore the future of ChatGPT’s memory capabilities.
Benefits for Current Users Adopting New Features
Users adopting the new features will experience several key benefits. Increased accuracy in responses due to the model’s enhanced context awareness will be a major improvement. The more nuanced conversational flow will create more natural and productive dialogues. This will enhance user experience, leading to more efficient task completion, improved creativity, and increased engagement with the model.
Potential Challenges and Limitations for Existing Users
One potential challenge for existing users is the adjustment period required to adapt to the new conversational style. Users accustomed to the previous model’s output might find the initial responses slightly different. Another potential limitation is the model’s ability to maintain context across extremely long or complex conversations. While the model demonstrates significant improvement in memory, there may be limits in retaining extensive amounts of detailed information.
Concluding Remarks
In conclusion, the nueva version memoria of the large language model showcases significant improvements in memory management, architecture, and training. These advancements result in a more powerful and user-friendly experience, with enhanced abilities to handle complex tasks and maintain context. The potential applications across various industries are vast, and the future looks bright for this powerful tool. Let’s now explore some frequently asked questions to further illuminate this impressive update.
Quick FAQs
What are the key improvements in memory management?
The new version employs advanced techniques for storing and retrieving information, leading to a more robust memory system. This allows the model to better retain context and previous interactions, leading to more accurate and personalized responses.
How will the new model affect existing users?
Existing users will experience a smoother and more intuitive interaction. Support resources will be available to guide them through the transition. The enhanced capabilities should provide a more beneficial experience overall.
What are some potential limitations of the new model?
While the new model is a significant improvement, potential limitations include the complexity of the underlying architecture, which could require more computational resources. Additionally, the evaluation process may not fully capture all possible use cases, and continued refinement may be necessary.
What industries might benefit most from this new model?
Industries such as customer service, education, and research stand to gain the most. The enhanced memory capabilities translate to more efficient and personalized interactions with customers, improved learning experiences, and faster analysis of complex data.